Hi @ricardomorenojr
Sorry for the delay, was occupied with stuff.
Disclaimer: You can go various ways with this. This is a way to do it. It’s always a tradeoff between like workflow performance, data set size, being comfortable working with certain nodes, etc.
I’ll illustrate two; one trough the Collection route and one through the Appender route. The final result is the same.
General:
I start with renaming the columns of both sources and also adding it as seperate column. This makes tracebility in your joiner and rule engine downstream a lot easier and gives more insights into the source that you are working with. The Column Rename Regex is usefull here whereby you can add a suffix to each column name, in this case I opt for PARF x
The subsequent joiner is pretty straightforward with a left outer join on the fields mentioned in your opening post as being the comparators. Also bringing the entire dataset from PARF B along.
By the looks of it you can find your way through a joiner, otherwise refer to the additional pointers of
@denisfi
I see in your workflow screenshot a common practise of Rule Engine + Row Filter. You can combine this when using a Rule Based Row Filter. Applying your logic in accordance with the transaction codes:
($Transaction Code_PARF A$ = "ACQU_DR" AND $Transaction Code_PARF B$ = "AP_CR") OR ($Transaction Code_PARF A$ = "ACQU_CR" AND $Transaction Code_PARF B$ = "AP_DR") => TRUE
Again, better understandable with the renamed columns and less prone to mistakes.
Here, you are at a junction. Since you want to have the entire rows of both PARF A and PARF B underneath eachother, I opt to handle them as entire group and create this structure.
Option 1:
Create a collection of data with all columns related to PARF A. Again, the renamed columns help here because I can apply a wildcard to capture all columns related to *PARF A
, KNIME automatically includes all eligable columns. It’s more conveniant to remove the aggregated columns from the input to avoid cluttering. Also designate that these are the aggregated values of A as per the table output.
- Repeat the process for B.
- Repeat the process to merge the A collection and B collection.
This will allow you to Ungroup them through the associated node. This places the A and B group rows underneath eachother.
Split the collection back into the original columns with a Split Collection Column node and a Column Rename to rename them according to your table.
Note: this assumes a fixed input format in terms of column order. If this changes, more work is needed to capture that.
This generates the final output that you are looking for in accordance with your provided Excel sheet.
Option 2:
Exactly the same principle, just different nodes. Main advantage: it “remembers” the spec of the columns which the Collection route is not very good with.
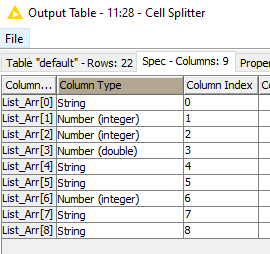
I’d say it depends on your use case if this is a gamechanger or not.
Option1 execution time: 44 ms
Option2 execution time: 43 ms
You can draw your own conclusions from that 
Note: can agree with your observation on the duplicate row filter suggestion. It needs the code comparison logic to accompony it but in larger dataset it can be used as a preselector to determine candidates subject to comparison.
See WF: Matching rows based on multiple criteria and from different categories.knwf (122.8 KB)
Hope this provides some inspiration!