Hi KNIMErs 
Interesting challenge this week!
I decided to approach this one as a clustering problem rather than purely image processing.
First of all, I read the image and used the -Splitter- node to split it into RGB Channels. I kept only the Red Channel and used the -Image to DataRow- node to convert the image to a collection of pixel intensity values. I then ungrouped the collection to organise the pixels into just one column of pixel intensities. The maximum image dimensions were then used to calculate the X and Y coordinates of each pixel using separate -Math Formula- nodes.
Using only the red channel is sufficient to discriminate between the pixels that represent the golf balls and those that represent the grass. Out of the three channels, the red channel is the one that discriminates the most between the grass and the balls. The reason for this is that the grass is green and the grass should be as dark as possible to differentiate them from the whiteness of the balls. The distribution of pixels per intensity can be seen in the histogram below:
The histogram clearly shows a bimodal distribution, where the left distribution corresponds to the grass pixels and the right distribution corresponds to the ball pixels. To discriminate between the grass and ball pixels I could have chosen the minimum height of the histogram as the threshold around position 130 where both distributions overlap. However, I have chosen a higher value of 200 to make sure that no pixels from the grass are retained. The choice of this threshold could also have been automated, but this could be the subject of another contest
The number of pixels was further reduced by randomly sampling 1000 rows from the remaining pixels.
The next step of the workflow determines the number of balls and their positions. Therefore, I applied the -Distance Matrix Calculate- node to the X and Y coordinates, followed by the -Hierarchical Clustering (DistMatrix)- node to generate a hierarchical cluster tree based on the ball pixels. Pixels from the same ball are closer together than pixels from other balls. To determine the optimum number of clusters (k), I used the -Parameter Optimization Loop Start- node starting from k=2 up to k=16 (double the number of golf balls in the image). The -Hierarchical Cluster Assigner- node assigns each pixel to a cluster based on the number of clusters in that iteration of the loop.
In order to save time, it is best to use this combination of nodes instead of just the -Hierarchical Clustering- node. This is because the -Hierarchical Clustering- node generates a tree each time it is run, however, the tree will always be the same, as it is independent of the number of clusters assigned to the data.
I have used the -Silhouette Coefficient- node inside the loop and then calculated the mean silhouette coefficient for each k value and plotted this using the -Line Plot (Labs)- node:
The plot clearly shows that the Optimum number of clusters is 8. I was very happy to see this, as there are 8 golf balls on the original image 
k=8 was therefore applied as the number of clusters in the final -Hierarchical Cluster Assigner- node:
The X and Y coordinates of each pixel plus the Mean X and Y coordinates of each cluster were plotted using the -Scatter Plot- node:
Again, I was very happy to see the result
The clusters are plotted in the same places as the golf balls on the image:
This proves that taking a sample of the data was sufficient enough to produce a good result!
In conclusion, the coordinates of the golf balls have been successfully determined and Caddie Tom would be able to use them to find where the golf balls are on his images. The method can be applied to different numbers of golf balls and different image sizes without the need to edit the workflow. However, the method could be refined since at the moment it detects anything with a white/off-white colour, so Tom may end up with a “chihuahua or muffin” situation on his hands 
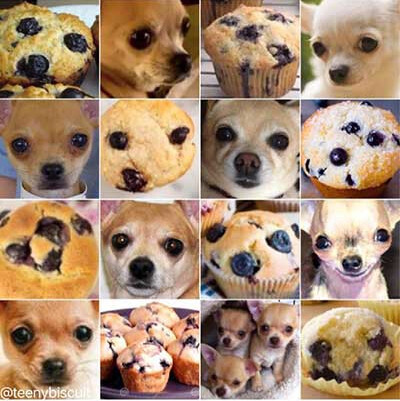
Here’s the link to my workflow on the hub:
Thanks @aworker for your supervision on this challenge!
Happy KNIMEing!!
Heather